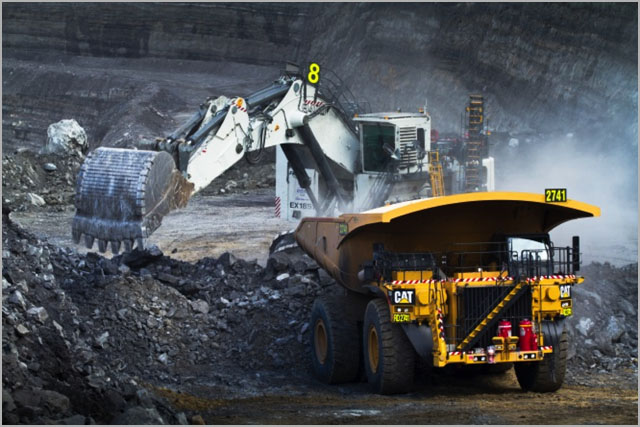
news Technology giant IBM last week revealed it had embarked on a new contract with construction and mining giant Thiess which is seeing Big Blue use so-called ‘Big Data’ techniques to improve the availability and operational productivity of Thiess’ mining equipment, initially focusing on the company’s mining haul trucks and excavators.
According to IBM, unlike traditionally data-intensive industries such as banking or telecommunications, which rely on advanced IT systems to drive operational performance, asset-intensive industries such as mining, have typically not invested as much operationally in IT systems.
Today, the company said in a statement issued last week, many organisations in natural resource industries rely heavily on either a ‘fix-it-when it-breaks’ approach or time-based scheduled maintenance techniques. These methods often result in “unnecessary downtime, premature component replacements, extra expense and lost production” and do not explicitly factor in an individual piece of equipment’s actual condition and performance capability.
However, IBM said, this trend was changing, as companies realised how IT could help them extract liquid, rocks and insights out of the ground. The increased deployment of machine and environmental sensors combined with new data collection methods is enabling the development of predictive machine maintenance analytics which can help increase equipment availability, lower production costs and provide greater operational flexibility.
According to IBM, the collaboration with Thiess has been integrating current and historical machine sensor data, along with maintenance and repair, operational, and environmental data to use as a basis for data-driven operational optimisation. Factors such as repair and inspection history, payload size, sensor-based component alerts, operator variability, weather, and ground conditions are being used to construct models which assess and predict the life of discrete components and the overall health of a piece of equipment.
“Analytics and modeling can offer great opportunities to improve our business, but we need to integrate them with our current processes in order to have a real bottom-line impact. Working with IBM to build a platform that feeds the models with the data we collect and then presents decision support information to our team in the field will allow us increase machine reliability, lower energy costs and emissions, and improve the overall efficiency and effectiveness of our business,” said Michael Wright, executive general manager Australian Mining, Thiess.
Early detection of even minor anomaly and malfunction patterns can be used, according to IBM, to predict the likelihood of component failures and other areas of risk. This will “dramatically” increase the uptime of the equipment and improve Thiess’ ability to manage the full life of discrete components, overall machine health and the deployment of limited maintenance resources.
Developing a unified predictive equipment and operational management system, according to IBM, requires finding a common connection between physical and computer scientists, who often operate with “different skill sets and goals”. The models used in this project bring together the physical and digital worlds by supplementing data-driven modeling that computer scientists tend to employ with information from engineers who have first-hand expertise about the mechanics of the equipment.
Predictive machine management differs from traditional machine management in several ways, according to IBM. First, it bases decisions about a machine’s maintenance and operation on the actual condition or health at that given time. Second, it has the ability to predict the health of a given machine far enough in the future to enable decision makers to execute correct actions such as adjusting production plans or ordering spare parts.
For example, in one mining scenario, several haul trucks that move coal may be reported to need maintenance, while at the same time a substantial order of product is due for delivery in 11 days. The predictive machine management system will be able to look at a variety of options for addressing this problem, and provide a decision maker with a model-based prediction that if the trucks are loaded no more than 85% of normal capacity and driven at no more than 80 percent of normal speed, the failure probability over the next 11 days would be minimal. This allows companies to avoid badly-timed and costly downtime at the sacrifice of only a minor, temporary decrease in throughput.
opinion/analysis
Pretty heavy stuff from IBM’s media release there. I hope readers were able to wade through it all :)
To my mind, what this project illustrates is the fact that new types of t echnologies are increasingly going to be embedded into all aspects of every type of business or organisation that exists. When you’re able to use technology to predict the lifecycle of the massive trucks and excavators that Thiess uses for mining, including the lifecycle of the discrete parts used, then you really can start to predict anything. I’m sure that this project cost Thiess a pretty penny — nothing IBM does ever comes cheap. I suspect that in future this kind of analytics will become increasingly commoditised as it is embedded everywhere and in everything.
Image credit: IBM/Thiess